Aim
The overarching aim of this project is to deliver real-world application-driven research into human-centric sensing techniques, by developing the applicable theory, algorithms, architectures and applications. Focusing on the problem of sensing data that lacks predictive quality, a thorough human-centric sensing framework, entailing user recruitment to quality-driven rewarding is expected.
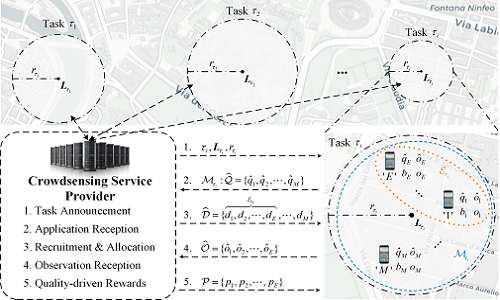
Funder
This project is part of the Data-Centric Engineering Programme jointly lled by the Lloyd’s Register Foundation and the Alan Turing Institute
Main Contributors
Dr Cong Zhao
Professor Shusen Yang
Prof. Julie A. McCann
Introduction
Compared to privately deployed sensor networks, human-centric sensing solutions like mobile crowdsensing systems can achieve more flexible and cost-effective economically. However, such systems suffer from unpredictable human behavior and privacy concerns, which affect the usability and accuracy of the systems. This project aims at developing a series of human-centric sensing solutions with data quality guarantees in privacy-preserving scenarios, which can be widely adopted to solve real-world problems with economical, large-scale and relatively accurate sensing requirements in applications such as urban information collection and environment monitoring.
Primary Research
User Incentivisation
Because of the self-interested nature of humans, potential participants will not take part in a sensing task unless there is considerable reward. However, since the difference in participants’ proficiencies in specific sensing tasks could be massive, in terms of knowledge, sensing capacity, and available time, the reward to each participant needs to be determined subtly as an effective stimulation. This project aims at modelling human-centric sensing tasks as competitive games among the service provider and multiple participants, and developing rational rewarding schemes to encourage active participation.
Truthful Reporting
Since privacy-preservation is critical to the practical implementation of human-centric sensing, the true identity and daily preference of human participators must not be revealed to the service provider. However, such a mandatory requirement casts serious challenge on the truthfulness of the information claimed by each participant, since there is no referable information about historical behaviors of the participants. This project aims at solving the untruthful reporting problem by developing strategy-proof rewarding methods which will theoretically ensure that truthful reporting is always the best choice for each participant.
Data Quality Evaluation and Enhancement
The result of human-centric sensing is determined on reported observations from an undetermined number of human participants. The evaluation of the quality of reported data is challenging since there is no referable ground truth. This project aims at discovering the sensing truth among reported observations using distributed data analysis methods considering the difference among each participant’s proficiency. Furthermore, adaptive methods based on reinforcement learning should also be developed to allow all participants to incrementally enhance their data quality for higher sensing profits.
Publications
- Zhao C, Yang S, McCann JA, 2019, On the data quality in privacy-preserving mobile crowdsensing systems with untruthful reporting, IEEE Transactions on Mobile Computing, Pages: 1-1, ISSN: 1536-1233
- Zhao C, Yang S, Yan P, et al., 2018, Data quality guarantee for credible caching device selection in mobile crowdsensing systems, IEEE Wireless Communications, Vol: 25, Pages: 58-64, ISSN: 1536-1284
- Zhao C, Yang S, Yang X, et al., 2016, Rapid, user-transparent, and trustworthy device pairing for D2D-enabled mobile crowdsourcing, IEEE Transactions on Mobile Computing, Pages: 2008-2022, ISSN: 1536-1233