Edge Intelligence & Meta-Learning
Data-centric Engineering broadly is the design, development and maintenance of engineered systems that are informed by data. AESE’s interest in this topic lies in the co-engineering of sensor-based systems with the physical systems under focus (water network, autonomous vehicle, smart city etc.). To make use of the data, beyond signal processing and statistics, we explore Edge-Intelligence, machine learning algorithms that can run on low-resources and low-power sensor networks. This has two purposes. Firstly, machine learning can inform a wireless sensor network to self-optimise, cope with failures, or ascertain if it is under attack. Secondly, edge learning can be used to pre-process the incoming sensor data to minimise data communications with the Cloud without the loss of information, and at the same time can provide side-advantages such as privacy preservation. To this end, we concentrate on decentralised and hierarchical approaches including Meta-Learning etc. that can operate under minimal training and which can carry out applications such as Nowcasting etc. Besides this, we also work on incentivisation to encourage heterogeneous edge device users to contribute high quality learning samples for effective distributed learning. Some of this work has been applied to tools to help visually impaired people.
Much of this work is in conjunction with the Alan Turing Institute, Data-Centric Engineering Programme.
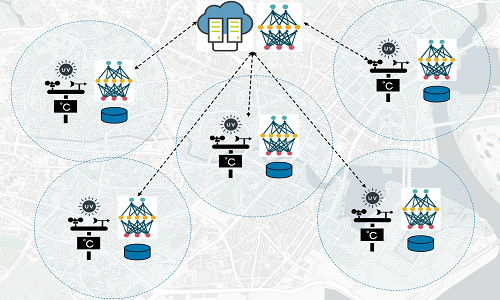
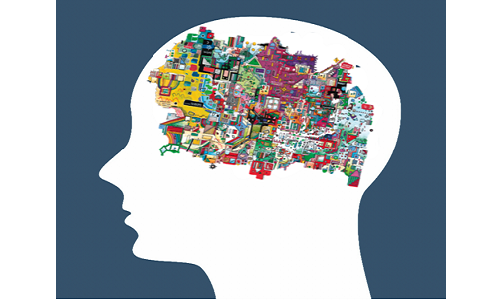
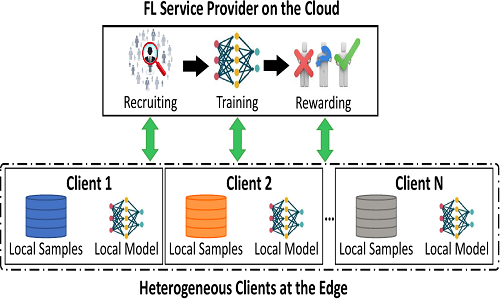