Computer Graphics Forum (Proc. of Pacific Graphics) 2023
Gilles Rainer ¹ ² Lewis Bridgeman ¹ Abhijeet Ghosh ¹ ²
Lumirithmic ¹
Imperial College London ²
Patent pending
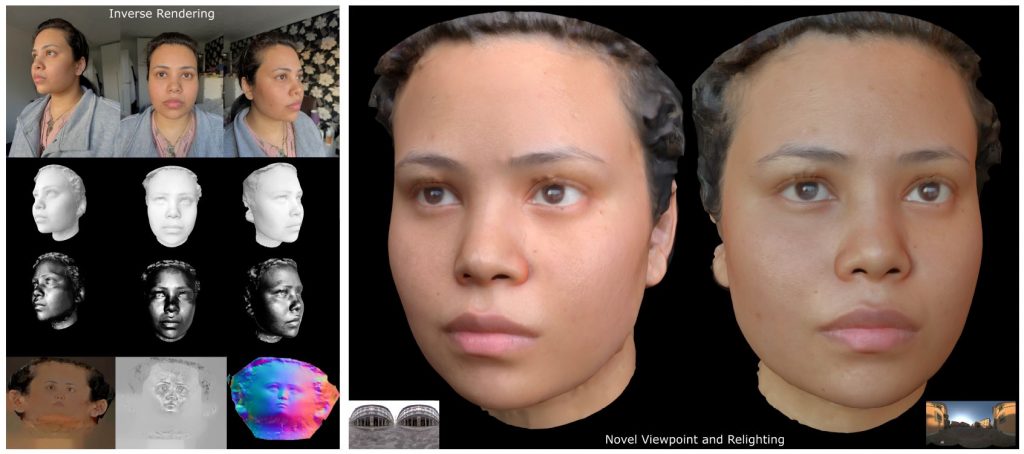
Fig. 1: From casual smartphone frames (left, top) in arbitrary unknown lighting, our inverse optimization uses tiny shading networks to efficiently factorize light transport (left, middle) and disentangle shading from explicit reflectance maps (left, bottom). The resulting asset (mesh & textures) can be used in common path-tracers to render novel viewpoints and lighting (right).
Abstract:
Given a set of unstructured photographs of a subject under unknown lighting, 3D geometry reconstruction is relatively easy, but reflectance estimation remains a challenge. This is because it requires disentangling lighting from reflectance in the ambiguous observations. Solutions exist leveraging statistical, data-driven priors to output plausible reflectance maps even in the under-constrained single-view, unknown lighting setting. We propose a very low-cost inverse optimization method that does not rely on data-driven priors, to obtain high-quality diffuse and specular, albedo and normal maps in the setting of multi-view unknown lighting. We introduce compact neural networks that learn the shading of a given scene by efficiently finding correlations in the appearance across the face. We jointly optimize the implicit global illumination of the scene in the networks with explicit diffuse and specular reflectance maps that can subsequently be used for physically-based rendering. We analyze the veracity of results on ground truth data, and demonstrate that our reflectance maps maintain more detail and greater personal identity than state-of-the-art deep learning and differentiable rendering methods.
Publication: Neural Shading Fields for Efficient Facial Inverse Rendering. Gilles Rainer, Lewis Bridgeman, and Abhijeet Ghosh. Computer Graphics Forum (Proc. Pacific Graphics), 42(7), 2023.